I gave my first tutorial @ PyData LA, 2019 on "Experimental ML with Holoviews/Geoviews + Pytorch". Here are my talk slides, video and jupyter notebook materials!
I participated in Geo4Good @ Google in Mtn View, CA! Check out some highlights of inspiring projects based on Google Earth Engine and Studio.
I'm a Computer Science PhD student at USC, working with Prof. Laurent Itti at iLab and ISI's VIMAL .
Before starting my PhD, I studied at MIT for my Bachelors and Masters in Electrical Engineering and Computer Science (EECS) with Minor in Mathematics.
During my Masters, I concentrated on Artificial Intelligence and worked under the joint guidance of Prof. Regina Barzilay and Dr. Julian Straub.
My main projects were (1) non-rigid image registration of mammograms for breast cancer detection, and (2) 3D reconstruction of human arms for efficient lymphedema screening. You can find out more about them
here.
Along the way, I worked at academic research labs at MIT (CSAIL, Media Lab, McGovern Institute) and INRIA (ILDA lab). I also interned in the industry, at a French robotics startup (Keecker), Apple (6-month COOP) and MathWorks (PhD Research Intern).
Research Interests
Geometric Fingerprints of Generative Models
Broadly, my research interest lies in understanding how complex, high-dimensional information-processing systems (e.g., human intelligence, modern generative models, collective behaviors like traffic patterns) behave and developing efficient algorithms to analyze their characteristics and traces in a principled way, from geometric perspectives.
Through this geometric understanding of their properties and internal mechanisms, I aim to improve their behaviors (e.g., steering away from degenerative patterns and biases while aligning to more proper values), by mechanistically intervening in their internal causal pathways. Of many such complex systems, my PhD research focuses on generative models and representation learning algorithms.
Currently, despite the rapid development of Generative A.I., there is still a big gap in our understanding and control over their designs and behaviors.
My aim is to fill this gap, by developing a formal framework and efficient algorithms that can represent, analyze and control the behaviors of generative models (e.g., Large Foundation Models (FMs)) in their high-dimensional spaces.
To this end, I approach these problems from geometric perspectives, and I am working on formalizing the theory of (generative) model behaviors using Differential Geometry and Riemannian Manifold.
Grounded on this theory, I also work to develop efficient algorithms that can extract geometric signatures of the behaviors and internal representations of large generative models (which live on much higher dimensional spaces than what the current geometric approach has been employed (e.g., 1D signals or 3D images) ),
in order to "fingerprint" and characterize them.
My recent work and extensions
I have started on this research endeavor through my work on fingerprinting generative models:
In our recent work published in CVPR 24, we proposed a useful theoretical framework to represent model behaviors on a data manifold (i.e., as vector fields on a data manifold constructed from real images).
We also formalized, for
the first time in the literature, the definition of “artifacts” and “fingerprints” of generative models in a geometric language, and proposed an effective attribution method to study and compare vision generative models.
(See more details here.)
I am excited to extend this work to a larger variety of Foundation Models, including LLMs and SoTA multimodal models, to study their fingerprints and proactively watermark the models.
I will also look into their internal mechanisms that cause these fingerprints and develop principled methods to intervene and control causal pathways that are responsible for different model behaviors (e.g., image artifacts by Vision GMs or hallucinations in LLMs).
I hope by researching these problems, I will contribute to a generalized theory of generative models and their internal mechanisms, which in turn help shape a safe and responsible integration of Generative A.I. into our society.
Research Thread 2
Another thread of my research lies at the intersection of representation learning and information theory, inspired by the way our perceptual system integrates multimodal sensory inputs via identifying invariant semantics. I am interested in understanding how the semantic information flows while processing observations from multiple modalities, using tools in deep learning and thermodynamic approaches to information flow.
My guiding question is,
How do we extract the shared semantics from observations expressed in vastly different representational forms (eg. images, sounds, written texts), and how do we create/actualize various forms of observations, starting from the semantics we want to communicate?
I approach this question from an information-processing point of view and am developing generative models with disentangled representation to jointly learn the analysis and synthesis processes of multimodal data. My most recent work introduces a generative model with adversarial training that learns spatial semantics from map tiles collected from diverse sources such as satellites, Google Street Map and custom rendering engines.
Currently, I am exploring different ways to understand our proposed model, in particular, by measuring semantic information and studying the flow of information between the latent partitions.
How we can quantify the amount of the shared semantic information captured by our model?
If we view each latent partition as a subsystem that constitutes a global system represented by the whole latent space, then we can view the the adversarial discriminator as a demon (like Maxwell's Demon) sitting at the boarder of the latent subsystems.
From this point of view, (how) does this adversary -- the demon sitting at the boarder of the content and style latent partitions -- achieve the non-equilibrium state of the semantic vs. domain-specific information by "sorting" or "organizing" the information into the correct partition as the training happens?
It's exciting to see how the ideas and tools in thermodynamics can help quantify and visualize this flow of semantic information in our model!
Click to expand
My journey started from noticing our own ability to (i) break down a complex observation into multiple chunks of smaller and abstract concepts and (ii) create a new idea by playing and recombining the conceptual building blocks. For instance, we can take a glimpse of this dance between abstraction and synthesis in a video of Picasso's live drawing:
More specifically, I'm intrigued by how seamlessly we extract a common semantic content from observations in vastly different representational forms (such as languages, images, gestures or sounds, and infinitely many forms within each modality), and reversely, how a semantic content can be expressed in various forms without losing its (overall) meaning
Hmm.. coarse-graining?.
My exploration starts with an hypothesis that a phenomena in reality, from which
our observations stem from, contains __semantic potentials__("potential" as in
_potential energy_ in Physics, or going further up the stream, as in Aristotle's
["Potentiality and actuality"](https://en.wikipedia.org/wiki/Potentiality_and_actuality)
This idea influenced Leibniz to develop the science of "dynamics".
Learning about such influence brings light into what Leibniz was struggling to hit the chord with ideas like 'power' and 'action'. Contemplate: Aristotle's "potential:actuality" vs. Leibniz's "power:action"
.)
I wonder,
- What is the relationship between _semantic information_
See [An Outline of a Theory of Semantic Information](https://dspace.mit.edu/bitstream/handle/1721.1/4821/RLE-TR-247-03150899.pdf?sequence=1), a [survey](https://plato.stanford.edu/entries/information-semantic/), and more recent work by [Kolchinsky and Wolpert](https://royalsocietypublishing.org/doi/10.1098/rsfs.2018.0041)
and _semantic potential_, i.e. the underlying _field_ from which individual observations are actualized into an instance of a natural phenomena, an event?
- What is the process -- or geometric constraints -- that leads the same semantics to different representational forms? Can we learn this process from multimodal data?
- What is the process through which an observer builds an understanding -- an internal representation -- of an event?
- What is the process through which we identify, extract and encode the invariant semantics from observations in diverse modalities?
- How can we define and measure the semantic information in our representations, efficiently?
- Can we model these processes by __learning a generative model from data collected from multiple modalities__?
---
## Specific Example
For instance, consider the following observations: $X^A$ is an image of a dog barking on the door, $X^B$ is a recording of a dog barking, and $X^C$ is a sentence written in the English language. The semantic content shared among the observations is "there is a dog barking", and each observation is the result of expressing (synm: rendering, stylizing) the semantic content into a form proper for its modality (ie. image, sound, written English language, respectively).
My question, at the representational level is, how do we identify the underlying, shared semantic contents from the information about domain-specific variations?
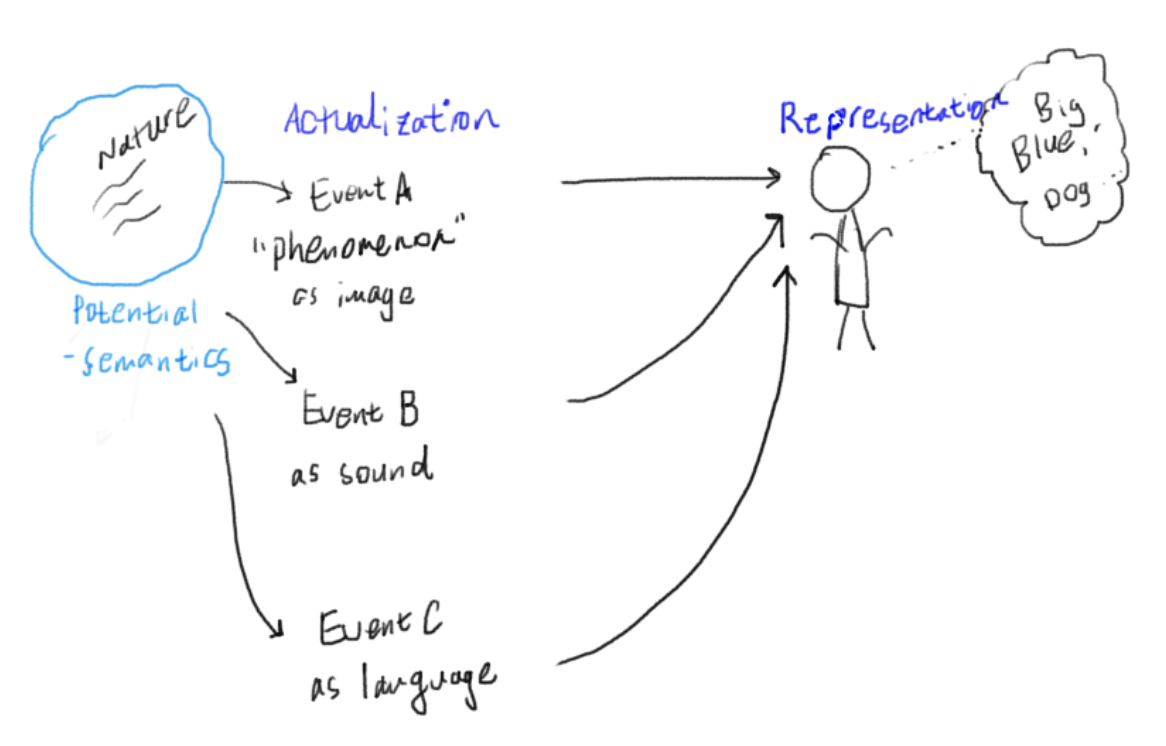
- How do we _identify_ what is the type of information that is invariant among observations from multiple domains?
- What discovery process goes into separating the shared contents (invariance across domain) from the domain-specifics?
- Can we use learning-based approaches to build a computational model of such process (i)more efficiently, (ii)by leveraging large amounts of data available?
Now let's flip the question and consider the process of synthesis. I start with a concept that I'd like to express and communicate. For example, I want to actualize the idea of "a dog barking at the door". If I ask you to express this content as an image, sounds, and an English sentence, what would be the process of such domain-specific actualization of a semantic information?
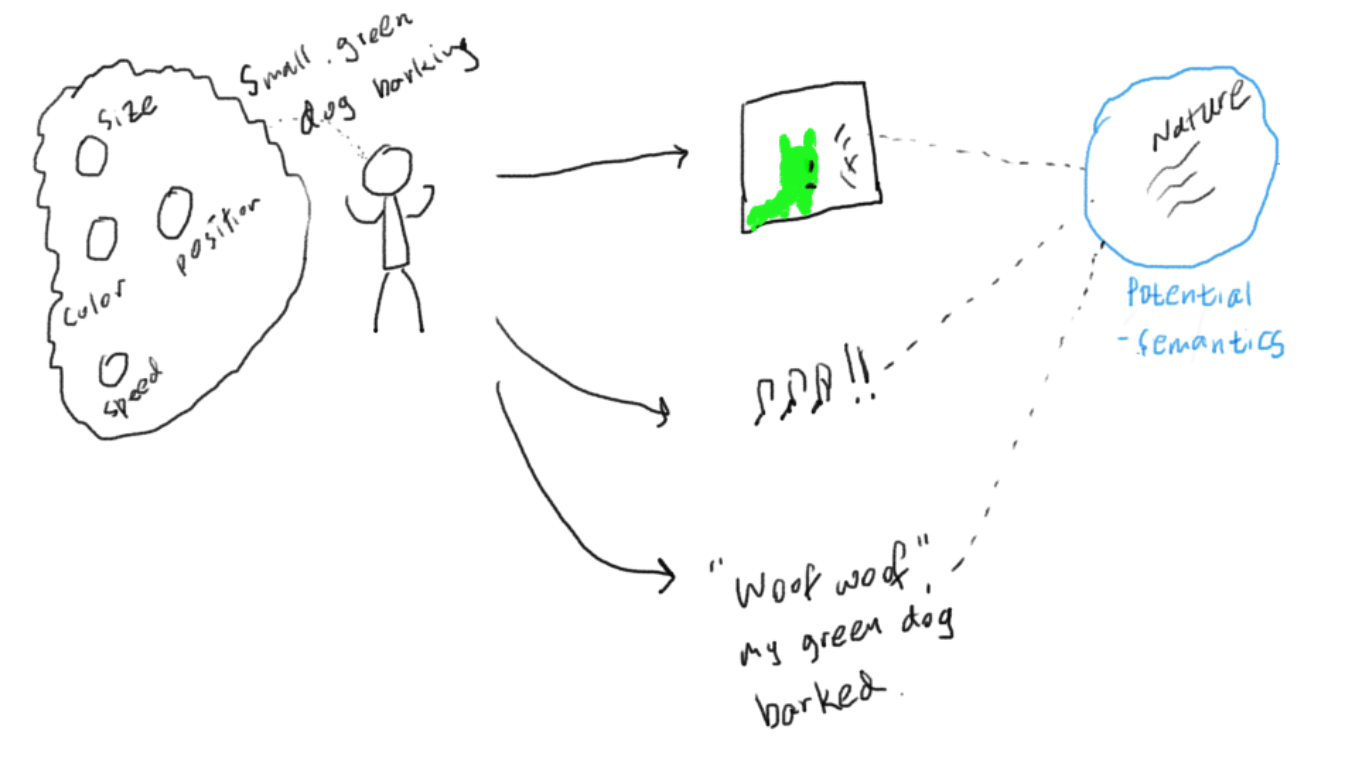
- What is the process of recombining the encoded representations to make better decisions, derive new conclusions? In particular, what is the underlying structure that defines each modality?
Geometry of a modality space: imposes geometric constraints that an instance must satisfy to be a valid observations of that modality
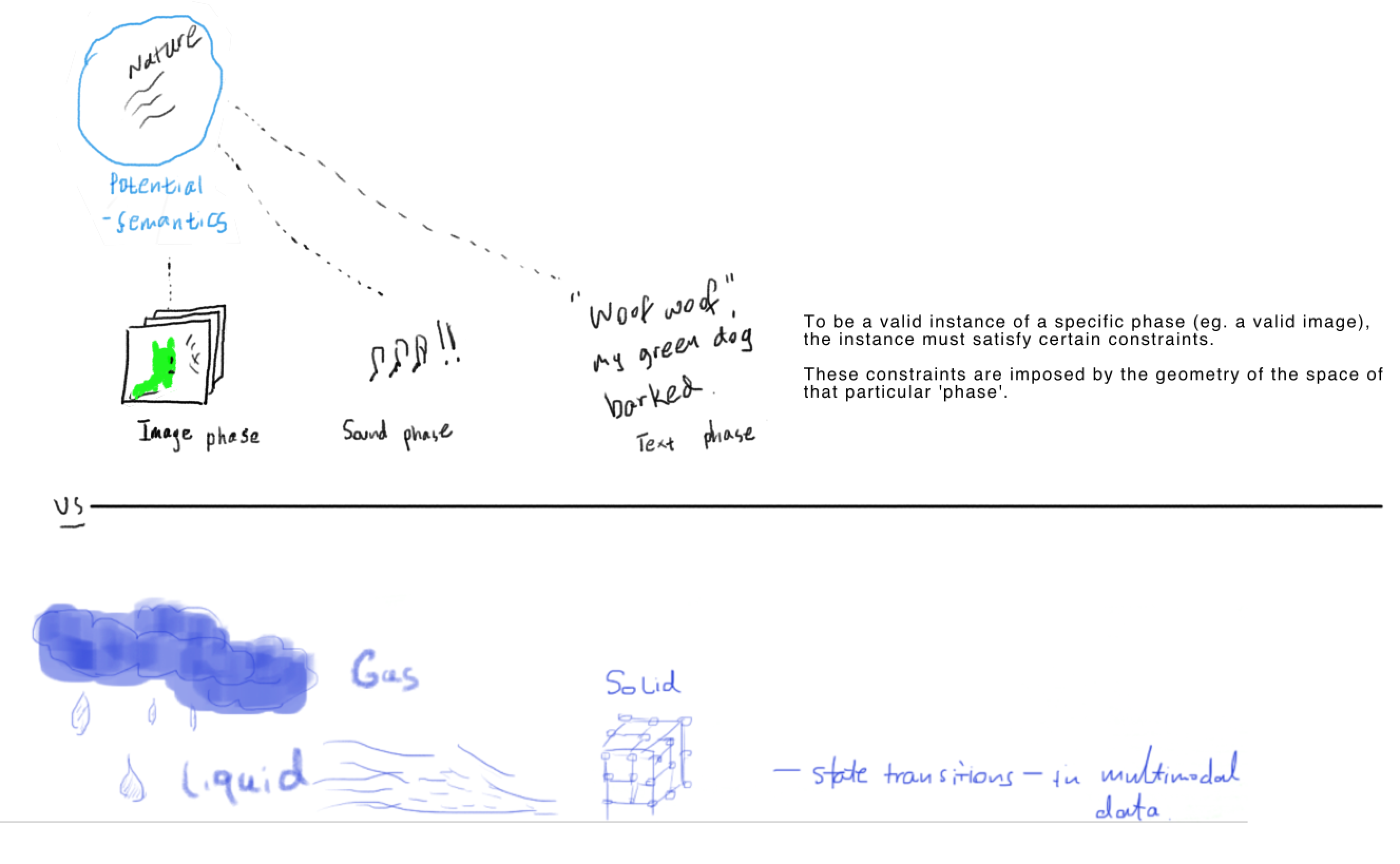
- E.g. an observation in an image form must satisfy a different set of geometric constraints than that in an acoustic form.
- Can we learn a model of such geometric rules via a generative model with neural networks?
The breakdown of main components of my questions looks as follows:
- Semantic information: Address a limitation of Shannon's theory of Information
The symmetry axiom of Shannon's entropy preserves the syntactic meaning in symbols, yet disregards their identities. See [ITTP2018](http://tuvalu.santafe.edu/~simon/it.pdf).
- Semantic potentials == a natural phenomena -- is this what "nature" is defined as?
- The process of actualizing semantic potentials/information to different modalities
- The process during which an observer builds an understanding of the actualized data point
- Geometry of modality space: what is the underlying geometry that defines an observation as a valid image vs. a valid human voice vs. a valid text?
---
## Research Statement
### Learning a generative model of multimodal representation
In pursuit of this computational model of understanding and generating multimodal data, I am developing generative models with disentangled representation to jointly learn the analysis and synthesis processes of complex, high-dimensional data (eg. satellite images, knowledge bases) with compact and “meaningful” representations.
I'm working with Prof. Wael Abd-Almageed at ISI's [VIMAL](https://vimal.isi.edu/),
focusing on various types of generative models for this goal.
My project with Prof. [Yao-Yi Chiang](https://spatial.usc.edu/team-view/yao-yi-chiang/) and Prof. [Craig Knoblock](https://usc-isi-i2.github.io/knoblock/) tackles this line of questions using geospatial data, and aims to learn spatial semantics from data that are collected from diverse sources (eg. satellites, Google Street Map, historical maps) and stored in diverse format (eg. images, graphs). This work has potential applications such as global-scale urban environment analysis, automated map synthesis and systems for monitoring environmental changes.
Within the domain of representation learning, I’m most interested in variational inference methods, especially recent developments in deep generative models such as variational autoencoders (VAEs) and the idea of adversarial training.
Using a VAE-variant model and adversarial training, I’m investigating how we can build a model that extracts invariance in a dataset of heterogeneous representations via VAEs and adversarial training. One of my current projects investigates this question in the domain of spatial informatics, using our new dataset of map tiles from diverse sources.
### Next itches
More about next steps...
Understanding adversary at the latent space from the perspectives of information flow and non-equilibrium achieved by the adversary, ie. the Maxwell's Demon at the gate that distinguishes the two latent partitions
GAN models are often described in the framework of min-max games between a generator and an adversary. In particular, there has been works making a connection between Nash Equilibrium and local minimum of the GAN's objective function. This connection motivates me to view my adversary (at the latent partitions) as an 'information sorter', like the Maxwell's Demon. The goal of this information sorter is to organize the semantic information into one latent partition, and the domain-specific information into the other latent partition, so that each partition (equivalent to a gas chamber in Maxwell's thought experiment) contains only its type of information. This approach will allow me to bring in computational tools from information theory and theromodynamics (flow of information) to understand how the adversarial information sorter actually achieves the partitioned latent space.
Evaluation of the disentangled partition requires a measure of semantic information
In order to evaluate how well our semantic latent space captures the semantic information in the inputs, we first need a well grounded _definition of the semantic information_, as well as _computational methods to efficiently compute_ the value.
Linking the discovered latent factors to external knowledge graph
---
## TMI: How much is Too Much Information?
More importantly, I'm practicing to:
- observe without being entangled in what is personal
- look at small thoughts carefully
- not to rush
- spend most of time on what matters most
- be gentle
- be slow
- be curious
- question
- relax in discomforts
- greet what is as what is
- stay open
> ‘Your act was unwise,’ I exclaimed, ‘as you see by the outcome.’
He solemnly eyed me.
‘When choosing the course of my action,’ said he,
‘I had not the outcome to guide me.’
- Ambrose Bierce
> Intention and attention.